- Think Ahead With AI
- Posts
- "The Next Frontier of AI: Inference Dominates" ๐
"The Next Frontier of AI: Inference Dominates" ๐
"Advancing AI Toward Precision Impact" ๐ฉ
Story Highlights: ๐ฏ
๐ค What is Model Inference?
๐ค How AI Inference Works
๐ค Challenges of Model Inference
๐ค AI at the edge
๐ค External vs. In-House AI Infrastructure
Who, What, When, Where, and Why: ๐
๐ Who: Professionals, business owners, and marketers interested in the future of AI inference.
๐ What: Exploring the significance of inference in AI, technical workings, challenges, and strategic decisions.
๐ When: In the rapidly evolving landscape of artificial intelligence.
๐ Where: Globally, wherever AI innovations are transforming industries.
๐ Why: To understand the pivotal role of inference in shaping AI's future and its implications for businesses.
What is Model Inference? ๐ก
Model inference is the process of utilizing a pre-trained artificial intelligence (AI) model to make predictions or decisions based on new, unseen data. This phase occurs after the model has been trained on a large dataset during the training phase.
During training, the AI model analyzes the dataset to learn patterns and relationships, effectively creating an internal representation of the problem it aims to solve.
This process involves adjusting the model's parameters iteratively to minimize errors and improve performance on the given task.
Once the training phase is complete and the model has been validated for its performance, it can be deployed for real-world applications.
In the inference phase, the pre-trained model receives new data inputs, which it processes through its learned internal representations.
Subsequently, the model generates predictions or decisions based on its understanding of the underlying patterns in the data.

In summary, model inference is the operational phase where a pre-trained AI model is used to analyze and make predictions on new data, leveraging the knowledge it gained during the training phase.
How AI Inference Works: ๐
Think of it as a streamlined process: data preprocessing, model execution, output generation, and post-processing. It's optimized for speed and efficiency, making it the engine behind AI's day-to-day operations.
๐ Data Preprocessing: Getting data ready for the AI model's consumption.
๐ Model Execution: The AI brain at work, making sense of data.
๐ Output Generation: The big reveal โ predictions emerge.
๐ Post-processing: Refining outputs for real-world applications.

How Model Inference works - credits of image to Nvidia Blog
Indeed, your example illustrates the concept of model inference effectively. ๐ฅ๐ฅ๐ฅ๐ฅ
Consider a natural language processing (NLP) model designed to understand and generate human-like text. During the training phase, the model is exposed to vast amounts of text data, ranging from literature and articles to social media posts and conversations. Through this exposure, the model learns the intricate nuances of language, such as grammar rules, semantics, and context.
Once the training phase is completed, the model transitions to the inference stage.
Here, it is presented with new text inputs and tasked with tasks like sentiment analysis, text summarization, or even generating creative writing.
For instance, when given a prompt like "Write a short story about a journey into space," the model utilizes its learned knowledge to craft a coherent narrative that fits the given theme.
In this scenario, the training phase involves processing extensive textual data to build the model's language understanding capabilities. On the other hand, inference represents the model's continuous application in real-world scenarios, where it analyzes, generates, or interprets text inputs based on its learned knowledge. While training is computationally intensive and time-consuming, inference is the model's ongoing utilization, which occurs frequently and at scale in various applications.
1. Personalized Movie Recommendations
Data: A streaming service records a user's viewing history, ratings, genres they typically watch, and how long they spend watching a movie or series.
Model: A machine learning system analyzes the user's viewing patterns and preferences. The algorithm might cluster users with similar tastes or use techniques like collaborative filtering.
Inference: When the user logs in, the model recommends new shows or movies tailored to their likely interests, encouraging longer engagement with the platform.
2. Email Spam Filtering
Data: Email systems analyze the contents of emails, including word choice, suspicious links or attachments, sender reputation, and whether similar emails have been flagged by other users.
Model: A machine learning model learns to identify patterns that commonly correspond to spam. It might be a decision tree, an algorithm that creates rules, or a neural network that finds complex relationships in the data.
Inference: As new emails arrive, the model scores them. Emails with high "spam" scores are automatically placed in the spam folder to protect the user.
Challenges of Model Inference: ๐
Despite its streamlined nature, inference comes with its own set of challenges. From performance and resource efficiency to security and transparency, navigating these hurdles is crucial for maximizing AI's potential.
AI at the Edge: ๐ฅ
Edge AI refers to the execution of AI algorithms directly on local devices, rather than relying solely on centralized cloud servers. Recent progress in edge computing has enabled this shift, allowing model inference to occur at the network's periphery. This development holds particular significance for applications such as autonomous vehicles.
Implementing models on edge devices offers numerous benefits.
Firstly, it minimizes latency by eliminating the need to transmit data to remote servers for processing.
Secondly, it optimizes bandwidth usage, as only the inference results are transmitted instead of the entire dataset.
Thirdly, it enhances privacy by ensuring that sensitive data remains on the device, thereby reducing the risk of unauthorized access or interception.
Edge computing is revolutionizing inference, enabling real-time processing on devices at the network's edge. It's faster, conserves bandwidth, and enhances privacy โ a game-changer for applications like self-driving cars.
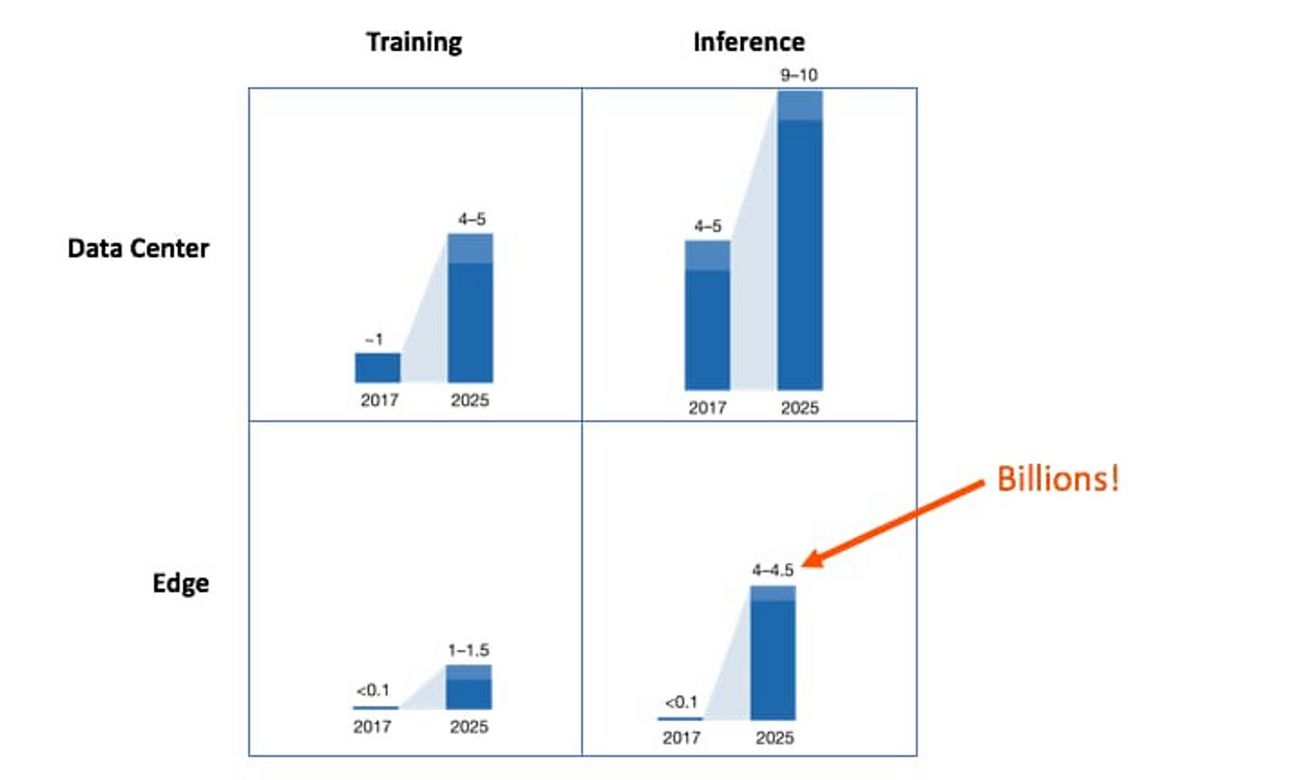
External vs. In-House AI Infrastructure: ๐ซ
The eternal dilemma: build in-house or leverage external services?
Both have pros and cons, from control and customization to time-to-value and ongoing costs. The choice depends on factors like workload scale and technical capabilities.
You've outlined the key considerations when deciding between building in-house AI infrastructure and leveraging external AI services:
Building In-House Infrastructure:
Pros:
Full control over the AI stack and deployment environment, allowing for customization to specific needs.
Potential for lower long-term costs, especially for high-volume inference workloads.
Ability to tailor hardware, software, and processes to meet unique requirements.
Cons:
Requires significant upfront investment and ongoing IT resources for development, maintenance, and scaling.
Longer time-to-value due to the need to build and configure infrastructure from scratch.
Responsibility for ensuring security, compliance, and reliability falls on the organization.
Using External AI Services:
Pros:
Faster time-to-value with pre-built, managed AI services that are ready to use.
Offload infrastructure management and scaling to the service provider, reducing operational burden.
Access to the latest AI hardware and software innovations without needing to invest in R&D.
Cons:
Ongoing subscription costs may be higher in the long term compared to building in-house infrastructure.
Limited control over the underlying infrastructure and deployment environment.
Dependency on the service provider's performance, reliability, and availability.
Ultimately, the decision depends on factors such as the scale and criticality of the inference workloads, the organization's technical capabilities, and its strategic goals.
Many organizations opt for a hybrid approach, leveraging external AI services for certain inference needs while building in-house solutions for mission-critical or high-volume use cases. This allows them to balance speed, flexibility, and cost-effectiveness while meeting their specific requirements.
Wrapping up: Trends in AI Infrastructure: ๐ฅณ
The future of AI infrastructure is inference-centric. While costs may stabilize, innovation will drive demand for powerful, scalable solutions.
Navigating this landscape is key to staying ahead in the AI race.
Why does it Matter to you and What Actions can you Take? ๐
๐ธ Stay updated on AI trends and advancements.
๐ธ Evaluate the suitability of AI inference for your business needs.
๐ธ Consider leveraging edge computing for real-time processing.
๐ธ Assess the pros and cons of building in-house infrastructure versus using external services.
๐ธ Invest in AI infrastructure to stay competitive in the evolving landscape.
Generative AI Tools ๐ง
๐๏ธ Sintra - A platform that automates repetitive tasks, freeing up your time to focus on the creative and strategic aspects of your work
๐ง Descript - All-in-one creative companion for effortless video and podcast editing with powerful AI features
๐ Grantable - AI-powered response drafting, Accelerated grant writing process, etc
๐ก Listening - Unique application that transforms academic papers into engaging audio experiences
๐ป Nuanced- Detect AI-generated images to protect the integrity and authenticity of your service.
News ๐ฐ
๐ Google Expands In-House Chip Efforts in Costly AI Battle
๐ Artificial Intelligenceโs โInsatiableโ Energy Needs Not Sustainable, Arm CEO Says
๐ Elon Musk and Jamie Dimonโs AI Predictions and What They Mean for the Future of Humanity
๐ Amazon CEO Touts AI Revolution While Committing to Cost Cuts
About Think Ahead With AI (TAWAI) ๐ค

Empower Your Journey With Generative AI.
"You're at the forefront of innovation. Dive into a world where AI isn't just a tool, but a transformative journey. Whether you're a budding entrepreneur, a seasoned professional, or a curious learner, we're here to guide you."
Founded with a vision to democratize Generative AI knowledge,
Think Ahead With AI is more than just a platform.
It's a movement.
Itโs a commitment.
Itโs a promise to bring AI within everyone's reach.
Together, we explore, innovate, and transform.
Our mission is to help marketers, coaches, professionals and business owners integrate Generative AI and use artificial intelligence to skyrocket their careers and businesses. ๐
TAWAI Newsletter By:

Sujata Ghosh
Gen. AI Explorer
โTAWAI is your trusted partner in navigating the AI Landscape!โ ๐ฎ๐ช